A First Look At Statcast’s Stolen Base Leaderboards
No, we didn’t use that photo of Rays legend Tommy Pham because he’s good at stealing bases. Or maybe he is. It’s complicated.
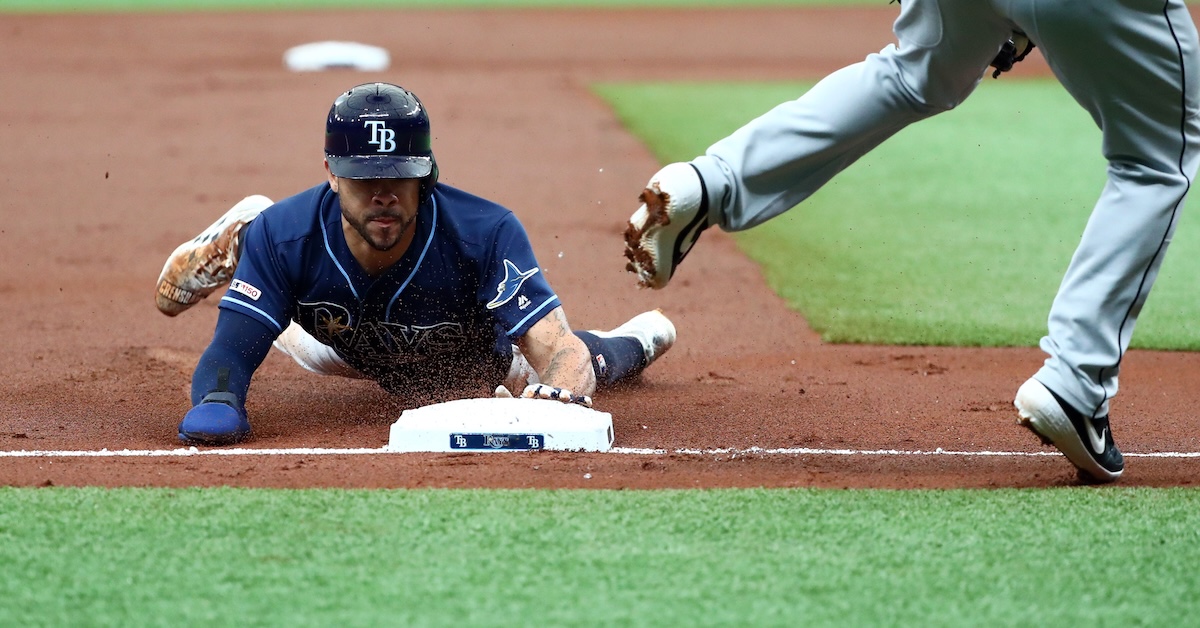
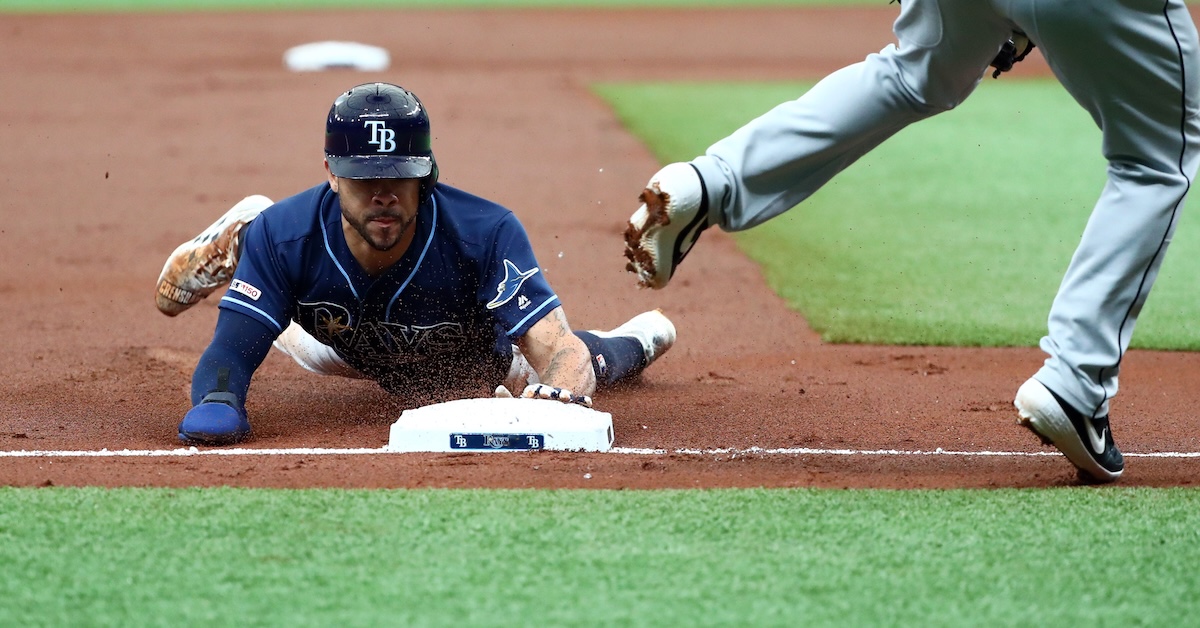
On Monday, Statcast took its the latest step toward the goal of consolidating all baseball data into one website so unimaginably massive that not even Joey Gallo’s batting average can escape its gravitational pull. Baseball Savant unveiled enhanced baserunning leaderboards, supplementing its leaderboard for extra bases taken with a separate leaderboard for basestealing, and also adding one that combines the two into an overall baserunning value leaderboard. (In a much quieter move that could end up being even more consequential for the super-duper data dorks in your life, Baseball Savant also introduced toggles for the first and second halves of the season into its search function.) I’ve spent the past couple days looking around at the numbers to see how this new information might change our understanding of the craft of baserunning, and I’d like to share my initial thoughts.
I think the big benefit of these data is they will teach us a lot about how particular players do what they do. MLB.com’s David Adler broke down some of the fun features of the new leaderboards, and if that’s your thing, there are indeed plenty of fun features to marvel at. If you surf around the leaderboard, you can see that on-base machine Juan Soto unsurprisingly led all players with 1,324 opportunities to steal a base this season. You can see that Mookie Betts gets excellent jumps when he’s stealing, traveling 6.1 feet between the moment of the pitcher’s first move and the moment of their release, the largest distance in the game. You can see just how anachronistic Lane Thomas’s 26-for-40 stolen base season really was.
However, so far I haven’t found anything that will revolutionize the way we see baserunning value as a whole. That’s not Statcast’s fault; it’s just that the data out there are already pretty good, and the value of a stolen base has been known for a while now. FanGraphs already uses Statcast’s extra bases taken numbers; they’re listed under XBR in the advanced tab of our batting leaderboard. We combine that number with wSB, (weighted stolen bases and caught stealing runs above average) to give you BsR, the total accounting of a player’s baserunning. Statcast is now showing you the same thing, resulting in an overall Baserunning Run Value metric, or BRV. Since 2016, 528 different players have made at least 1,000 plate appearances. The correlation coefficient between their BsR and their BRV, is .99, or very nearly identical. The correlation between BRV and Baseball Prospectus’s Deserved Runs on Bases metric is .91. So when you look at the overall numbers, the three existing metrics are similar enough to be interchangeable.
If we look just at the new data for runs created on stolen base attempts, Statcast’s new metric and our wSB still have a correlation coefficient of .94. They’ll obviously be less consistent over any one season, but over our nine-year sample, the numbers are more or less in lockstep. There’s only one player whose basestealing has been worth at least 2.5 runs according to one system, but cost his team runs according to the other system. Ladies and gentlemen, meet the enigma known as Tommy Pham.
Somehow, our numbers indicate that Pham’s basestealing has been worth 5.9 runs, while Statcast has him at -3.0 runs. That discrepancy has some extremely satisfying symmetry: In this 528-player sample, our numbers have Pham ranked 50th from the top, but Statcast’s numbers have him ranked 50th from the bottom. How could there be such a wild divergence when the overall numbers are so similar? And if that kind of divergence is possible, how is it that it’s only happening for one player?
You can read about how we calculate wSB in our library, but the short version is that we calculate how many runs each player creates per opportunity for a steal, then we compare it to the league average. Statcast does the same thing, but they’re breaking the data down more granularly, taking into account the situation and the expected success rate “based on the success probability of all those stolen base opportunities.” If you click on any player, you can see how many runs they’re credited with on their own – the standard 0.2 runs per stolen base and -0.45 runs for getting thrown out – along with runs awarded based on the pitcher, catcher, and fielder. Pham’s numbers don’t add up the way I expected them to – they add up to -0.68 runner runs, -0.50 based on the pitchers, -0.60 based on the catchers, and -4.20 based on the fielders, for a grand total of -5.98, and not the -3.0 overall number he’s credited with – so I’m clearly doing something wrong here.
As for which factors are being taken into account, I don’t know that either, but it’s not hard to guess. Does the pitcher control the running game poorly? If so, you might get less credit for a stolen base, or you might get docked even more for not stealing. As a result, a player could conceivably game the system by being on the back end of double steals, stealing in first-and-third situations, or just picking other really good spots where the chance of being thrown out is extremely unlikely. Our numbers would just credit them for taking the extra bases, whereas Statcast might dock them a bit because their success rate wasn’t that much higher than you’d expect based on the situation. Like I said, these are just guesses, and even if some are correct, I’m not sure which number I’d trust more. Presumably, the difficulty of a player’s opportunities will even out over time, but Pham’s star turn as an outlier indicates that won’t always be the case.
I’m not done exploring the data, and there are sorts of splits to examine. For example, if you pull the Statcast data into a CSV, you can see that they break the data for extra bases taken down into three categories with extremely catchy names: Swipes, Snipes, and Freezes. Here’s hoping those catch on around the game. But as is so often the case, Statcast’s big benefit is understanding probabilities in a new way. I’m not sure how granular it gets, and I’m not sure how much context would be too much. Say you steal a base on a curveball in the dirt. Should you lose some credit because that’s an easy pitch to steal on, or should you gain some credit because you wisely picked an easy pitch to steal on? Presumably, things balance out over a large enough sample size, so maybe a simpler approach is best.
Regardless, it’s fun to know, as Adler noted, that Elly De La Cruz and Bobby Witt Jr. both get particularly bad jumps, which makes sense because they’re so fast that they’ve never had to bother getting good jumps. If I were coaching the Reds or the Royals, I’d definitely be thrilled to know that there’s a such a simple way that my star player could improve his game. So far, that’s my biggest takeaway. Depending on the situation, a stolen base is just a stolen base, but by factoring in the ability of the pitcher and catcher to hold runners, the lead, the pitch, the jump, the throw, the tag, the firehose of Statcast data can paint a picture about the degree of difficulty. I’m sure there will be actionable data here, but for now, the numbers help tell the story in a new way.